
Topic models for comparative summarization
This paper aims to sum up our work in the area of comparative summarization and to present our results. The focus of comparative summarization is the analysis of input documents and the creation of summaries which depict the most significant differences in them. We experiment with two well known methods – Latent Semantic Analysis and Latent Dirichlet Allocation – to obtain the latent topics of documents. These topics can be compared and thus we can learn the main factual differences and select the most significant sentences into the output summaries. Our algorithms are briefly explained in section 2 and their evaluation on the TAC 2011 dataset with the ROUGE toolkit is then presented in section 3.
Keywords: comparative summarization, latent semantic analysis, la- tent dirichlet allocation, topic model, rouge
Year: 2013

Authors of this publication:
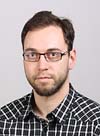
Michal Campr
E-mail: mcampr@kiv.zcu.cz
WWW: http://home.zcu.cz/~mcampr/
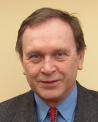
Karel Ježek
Phone: +420 377632475
E-mail: jezek_ka@kiv.zcu.cz
WWW: https://cs.wikipedia.org/wiki/Karel_Je%C5%BEek_(informatik)
Related Projects:

Automatic Text Summarisation | |
Authors: | Josef Steinberger, Karel Ježek, Michal Campr, Jiřà Hynek |
Desc.: | Automatic text summarisation using various text mining methods, mainly Latent Semantic Analysis (LSA). |