
Scoring System for Quantifying the Privacy in Re-Identification of Tabular Datasets
This study introduces a System for Calculating Open Data Re-identification Risk (SCORR), a framework for quantifying privacy risks in tabular datasets. SCORR extends conventional metrics such as k-anonymity, l-diversity, and t-closeness with novel extended metrics, including uniqueness-only risk, uniformity-only risk, correlation-only risk, and Markov Model risk, to identify a broader range of re-identification threats. It efficiently analyses event-level and person-level datasets with categorical and numerical attributes. Experimental evaluations were conducted on three publicly available datasets: OULAD, HID, and Adult, across multiple anonymisation levels. The results indicate that higher anonymisation levels do not always proportionally enhance privacy. While stronger generalisation improves k-anonymity, l-diversity and t-closeness vary significantly across datasets. Uniqueness-only and uniformity-only risk decreased with anonymisation, whereas correlation-only risk remained high. Meanwhile, Markov Model risk consistently remained high, indicating little to no improvement regardless of the anonymisation level. Scalability analysis revealed that conventional metrics and Uniqueness-only risk incurred minimal computational overhead, remaining independent of dataset size. However, correlation-only and uniformity-only risk required significantly more processing time, while Markov Model risk incurred the highest computational cost. Despite this, all metrics remained unaffected by the number of quasi-identifiers, except t-closeness, which scaled linearly beyond a certain threshold. A usability evaluation comparing SCORR with the freely available ARX Tool showed that SCORR reduced the number of user interactions required for risk analysis by 59.38%, offering a more streamlined and efficient process. These results confirm SCORR’s effectiveness in helping data custodians balance privacy protection and data utility, advancing privacy risk assessment beyond existing tools.
You will find the article also on the publisher's website.
Keywords: Anonymization; privacy; re-identification risk; GDPR; uniqueness; uniformity; correlation; open data
Year: 2025

Authors of this publication:
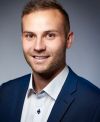
Michael Heigl
E-mail: heigl@kiv.zcu.cz
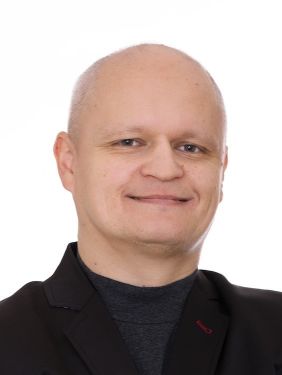
Dalibor Fiala
Phone: +420 377 63 2429
E-mail: dalfia@kiv.zcu.cz
WWW: http://www.kiv.zcu.cz/~dalfia/