
Knowledge-poor Multilingual Sentence Compression
We present a feature-based method for sentence compression. Firstly, a summary is created by our summarization method based on latent semantic analysis. The compression approach then removes unimportant clauses from the summary sentences. For each sentence a set of its possible compressed forms (compression candidates) is created. The candidates are then classified using 8 proposed features into two classes: in the first class there are candidates in which the important information was removed by compression and in the second class the information was still contained. The shortest candidate from the latter group substitutes the full sentence in the summary. The features are knowledge-poor which enables them to work with whatever language and the method can be easily extended by other features.
Keywords: Text summarization, sentence compression
Year: 2007

Authors of this publication:
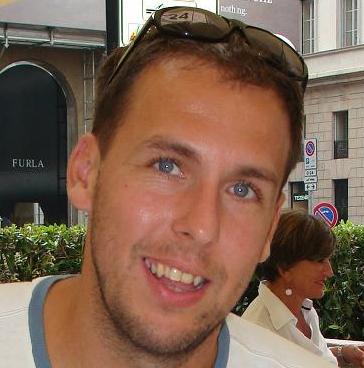
Josef Steinberger
E-mail: jstein@kiv.zcu.cz
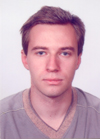
Roman Tesař
Phone: +420 377632479
E-mail: roman.tesar@gmail.com
WWW: http://www.sweb.cz/romant1/CV.pdf
Related Projects:

Automatic Text Summarisation | |
Authors: | Josef Steinberger, Karel Ježek, Michal Campr, Jiří Hynek |
Desc.: | Automatic text summarisation using various text mining methods, mainly Latent Semantic Analysis (LSA). |