
Update Summarization Based on Novel Topic Distribution
This paper deals with our recent research in text summarization.The field has moved from multi-document summarizationto update summarization. When producing an updatesummary of a set of topic-related documents the summarizerassumes prior knowledge of the reader determined by a setof older documents of the same topic. The update summarizerthus must solve a novelty vs. redundancy problem. Wedescribe the development of our summarizer which is basedon Iterative Residual Rescaling (IRR) that creates the latentsemantic space of a set of documents under consideration.IRR generalizes Singular Value Decomposition (SVD) andenables to control the influence of major and minor topicsin the latent space. Our sentence-extractive summarizationmethod computes the redundancy, novelty and significanceof each topic. These values are finally used in the sentenceselection process. The sentence selection component preventsinner summary redundancy. The results of our participationin TAC evaluation seem to be promising.
Keywords: Multi-Document Summarization, Update Summarization, Summarization Evaluation, Text Analysis Conference
Year: 2009

Authors of this publication:
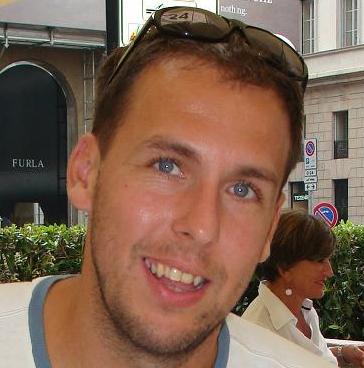
Josef Steinberger
E-mail: jstein@kiv.zcu.cz
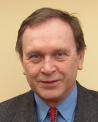
Karel Ježek
Phone: +420 377632475
E-mail: jezek_ka@kiv.zcu.cz
WWW: https://cs.wikipedia.org/wiki/Karel_Je%C5%BEek_(informatik)
Related Projects:

Automatic Text Summarisation | |
Authors: | Josef Steinberger, Karel Ježek, Michal Campr, Jiří Hynek |
Desc.: | Automatic text summarisation using various text mining methods, mainly Latent Semantic Analysis (LSA). |