
Evaluation of Automatic Speaker Recognition Approaches
This paper deals with automatic speaker recognition in Czech. We focus here on context independent speaker recognition with a closed set of speakers. To the best of our knowledge, there is no comparative study about different speaker recognition approaches on the Czech language. The main goal of this paper is thus to evaluate and compare several parametrization/classification methods in order to build an efficient Czech speaker recognition system. All experiments are performed on a Czech speaker corpus that contains approximately half one hour of speech from ten Czech native speakers. Four parameterizations, which are mentioned in other studies as particularly successful for the speaker recognition task, are compared: Mel Frequency Cepstral Coefficients (MFCC),Perceptual Linear Prediction Coefficients (PLPC), Linear Prediction Reflection Coefficients (LPREFC) and Linear Prediction Cepstral Coefficients (LPCEPSTRA). Two classifiers are compared: Hidden Markov Models (HMMs) and Multi-Layer Perceptron (MLP). In this work, we further study the impact of varying sizes of training corpus and test sentence on the recognition accuracy for different parametrizations and classifiers. For instance, we experimentally found that the recognition is still very accurate for test utterances as short as two seconds. The best recognition accuracy is obtained with LPCEPSTRA/LPREFC parametrizations and HMM classifier.
Keywords: automatic speaker recognition, Czech language, Hidden Markov Models (HMMs), Multi-Layer Perceptron (MLP), speech parameterization
Year: 2009

Authors of this publication:
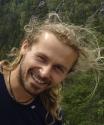
Pavel Král
Phone: +420 377 632 454
E-mail: pkral@kiv,zcu.cz
WWW: http://home.zcu.cz/~pkral/