
Unsupervised Feature Selection for Outlier Detection on Streaming Data to Enhance Network Security
Over the past couple of years, machine learning methods—especially the outlier detection ones—have anchored in the cybersecurity field to detect network-based anomalies rooted in novel attack patterns. However, the ubiquity of massive continuously generated data streams poses an enormous challenge to efficient detection schemes and demands fast, memory-constrained online algorithms that are capable to deal with concept drifts. Feature selection plays an important role when it comes to improve outlier detection in terms of identifying noisy data that contain irrelevant or redundant features. State-of-the-art work either focuses on unsupervised feature selection for data streams or (offline) outlier detection. Substantial requirements to combine both fields are derived and compared with existing approaches. The comprehensive review reveals a research gap in unsupervised feature selection for the improvement of outlier detection methods in data streams. Thus, a novel algorithm for Unsupervised Feature Selection for Streaming Outlier Detection, denoted as UFSSOD, will be proposed, which is able to perform unsupervised feature selection for the purpose of outlier detection on streaming data. Furthermore, it is able to determine the amount of top-performing features by clustering their score values. A generic concept that shows two application scenarios of UFSSOD in conjunction with off-the-shell online outlier detection algorithms has been derived. Extensive experiments have shown that a promising feature selection mechanism for streaming data is not applicable in the field of outlier detection. Moreover, UFSSOD, as an online capable algorithm, yields comparable results to a state-of-the-art offline method trimmed for outlier detection.
You will find the article also on the publisher's website.
Keywords: feature selection; outlier detection; intrusion detection; network security; machine learning; online learning; unsupervised learning; streaming data
Year: 2021

Authors of this publication:
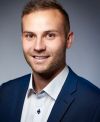
Michael Heigl
E-mail: heigl@kiv.zcu.cz
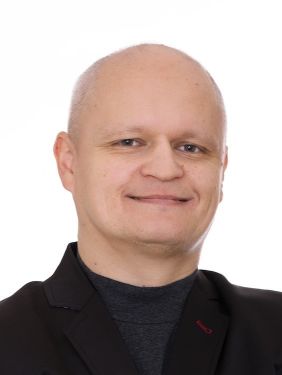
Dalibor Fiala
Phone: +420 377 63 2429
E-mail: dalfia@kiv.zcu.cz
WWW: http://www.kiv.zcu.cz/~dalfia/
Related Projects:

Data Mining for Computer Networks Security | |
Authors: | Michael Heigl, Laurin Doerr, Dalibor Fiala |
Desc.: | Novel data mining methods for the enhancement of computer networks security using advanced outlier detection techniques on streaming data are investigated. |